Today, many machine learning algorithms are based on patterns and statistics. That means that without a lot of training data these algorithms are not that intelligent or effective.
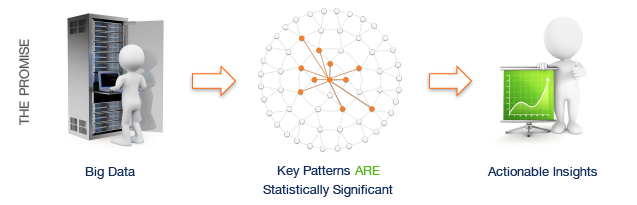
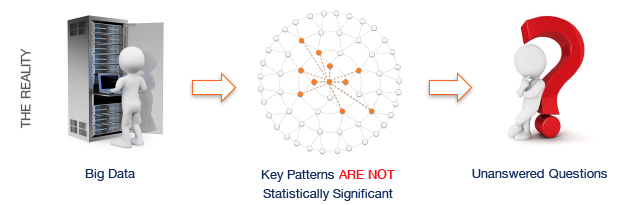
People often think that having a lot of data is a good thing. The challenge is, without a large number of training data machines don’t know what to look for, or how to structure data; while statistical relationships only reveal general insights.
How can we go from general insights to granular level insights that can affect the bottom line? There are a number of approaches to address these issues.
The most popular approach is acquiring the needed training data, indexes, and libraries from other companies. Google provides some training sets that are popularly used today. Those training sets are designed to solve the most popular issues, such as labeling images, but rarely address the unique data challenges of individual enterprises.
Results of classifying data based on patterns (image source: imgur.com):
Manually labeling data and manually building taxonomies and ontologies are other popular approaches. They are extremely time-consuming and require domain expertise that many data scientists lack.
Logyc took another approach to build machine learning solutions and discovering actionable insights. We designed a human-augmented machine learning technology that leverages the knowledge available within the enterprise, to compensate for the context lost during the digitization process. Logyc makes capturing implied and statistically insignificant insights feasible, something that is rarely done by others due to the scalability issues that we were able to resolve.
While there are different approaches to becoming a data-driven company, one should always consider the limitations of particular technology relative to one’s unique data and business challenges. Starting with the question, how can data science transform your enterprise and your industry?